Revolutionizing IT Services with Spam Detection Using Machine Learning
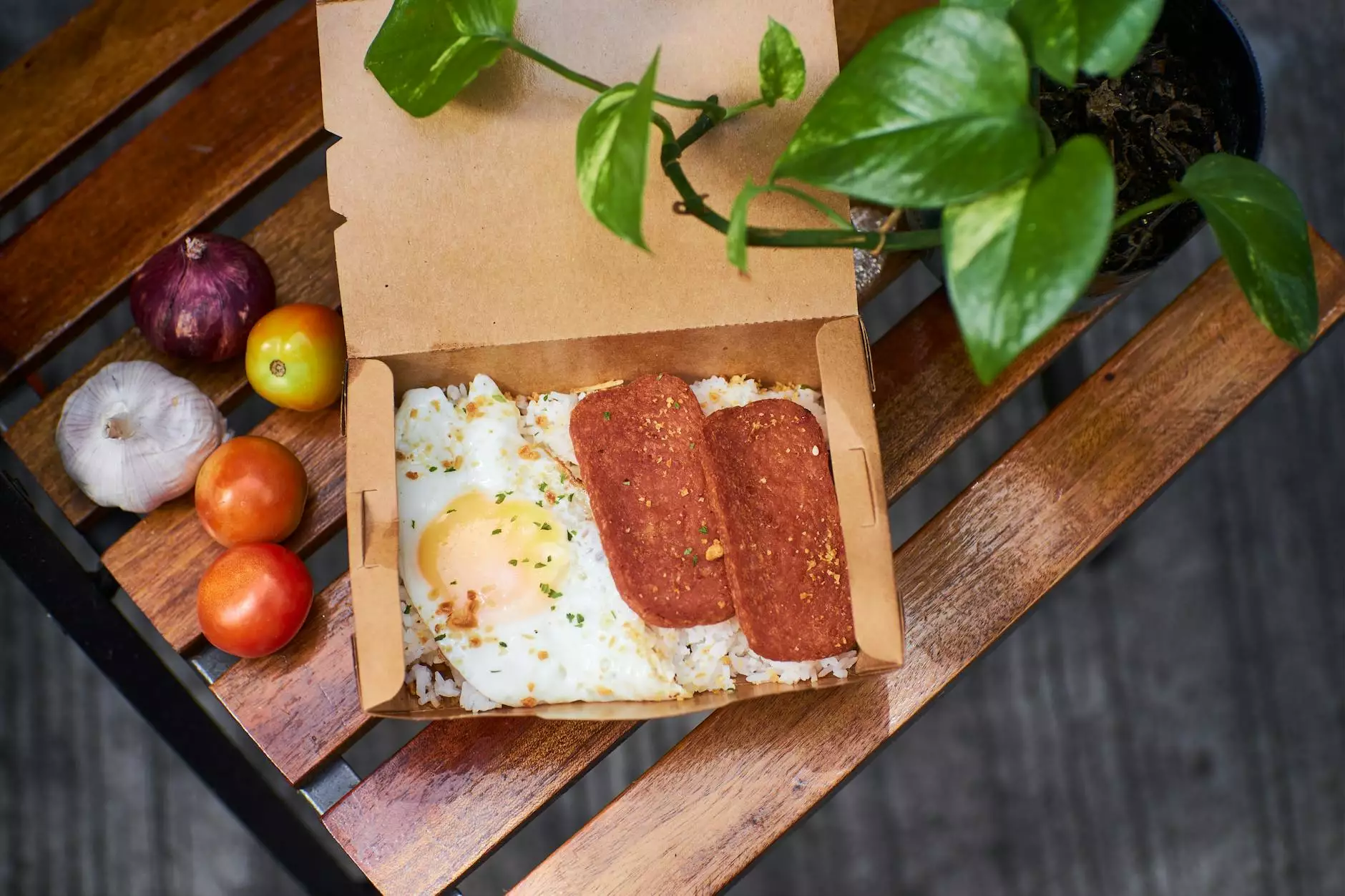
In the ever-evolving landscape of technology, spam detection has become a critical component for businesses aiming to maintain a secure and efficient digital ecosystem. With a surge in online threats and cybersecurity attacks, integrating spam detection using machine learning into business operations has proven to be a game-changer. This article delves deep into the mechanisms of spam detection, its importance for IT services and computer repair, and how it enhances security systems.
Understanding Spam and Its Impact on Businesses
Spam refers to unsolicited and often irrelevant messages sent over the internet, typically for advertising purpose. These messages can infiltrate emails, social media platforms, and other communication channels, leading to several detrimental effects:
- Decreased Productivity: Employees spend valuable time filtering spam emails and messages.
- Security Risks: Spam can carry phishing links or malware, compromising business data.
- Reputation Damage: Continual spam presence can harm a brand's reputation, leading to distrust from customers.
The need for robust spam detection systems is more critical than ever. This is where machine learning technology steps in, offering sophisticated solutions to the spam dilemma.
What is Machine Learning?
Machine learning is a subset of artificial intelligence that enables systems to learn and improve from experience without being explicitly programmed. It allows algorithms to analyze vast amounts of data, identify patterns, and make decisions based on the information gleaned. In the realm of spam detection using machine learning, algorithms can be trained to discern between legitimate messages and spam.
How Machine Learning Enhances Spam Detection
The traditional spam detection methods relied heavily on keyword filters and blacklists, which often failed to adapt to new spam tactics. Machine learning offers advanced techniques that significantly improve accuracy and efficiency:
1. Predictive Modeling
Machine learning models can be trained using historical data on spam emails and legitimate communications. By identifying common characteristics of spam, these models predict the likelihood of new messages being spam.
2. Natural Language Processing (NLP)
NLP enables machines to understand human language. By employing NLP techniques, spam detection systems can analyze the context, structure, and sentiment of messages to better identify spam content.
3. Feature Extraction
The process of isolating various elements from messages such as sender information, links, and formatting enables more granular analysis. This method helps in building comprehensive profiles that distinguish between spam and legitimate emails.
4. Continuous Learning
One of the most promising aspects of machine learning is its ability to learn continuously. As new spam techniques emerge, machine learning algorithms adapt and evolve to maintain their effectiveness. This ensures businesses are protected against the ever-changing spam landscape.
The Role of Spam Detection in IT Services
In the world of IT services and computer repair, spam detection is a linchpin that supports an array of operational processes:
1. Protecting Sensitive Data
With the increase in cyberattacks, safeguarding sensitive information from spam-related threats is paramount. Effective spam detection systems prevent unauthorized access to critical data by filtering out harmful messages before they can do damage.
2. Enhancing Customer Trust
A business that effectively manages spam is likely to inspire greater trust among its customers. When clients see that their communications are handled securely, their confidence in your IT services escalates.
3. Streamlining Operations
By automating spam detection, businesses can streamline their communication processes. This allows IT teams to focus on higher-level tasks, improving overall operational efficiency.
Implementing Machine Learning for Spam Detection
Integrating machine learning into spam detection requires a carefully crafted strategy. Below are key steps for businesses looking to adopt this technology:
1. Data Collection
Start with gathering a substantial amount of data sets, including both spam and non-spam emails. This dataset will serve as the foundational training material for your machine learning models.
2. Model Selection
Choose the appropriate machine learning model that best fits your data and business needs. Popular models for spam detection include:
- Naive Bayes Classifier
- Support Vector Machines
- Random Forest
3. Training the Model
Training involves feeding your chosen model the previously collected data so it can learn to identify patterns associated with spam and legitimate emails. The more diverse and extensive your dataset, the better your model will perform.
4. Testing and Validation
After training, the model needs to be rigorously tested to ensure its accuracy and reliability. This phase includes validating the model against a new dataset to gauge its performance in real-world scenarios.
5. Continuous Improvement
Post-deployment, continuous monitoring and improvement are essential. As spam tactics evolve, so must your model. Regularly update your datasets and retrain your model to maintain high levels of detection accuracy.
Short- and Long-Term Benefits of Machine Learning in Spam Detection
The implementation of spam detection using machine learning not only addresses immediate spam-related issues but also contributes to long-term benefits across your business operations:
Short-Term Benefits
- Immediate Reduction in Spam: Enhanced accuracy in filtering out spam right from the start.
- Faster Response Times: IT support can respond rapidly to genuine concerns without sifting through spam.
- Improved User Experience: Employees can focus on meaningful communications, thereby increasing satisfaction and productivity.
Long-Term Benefits
- Robust Security Framework: Building a strong foundation against future assaults and threats.
- Cost Efficiency: Reducing the time and resources spent on managing spam-related investigations.
- Stronger Brand Image: An organization that prioritizes user security portrays professionalism and reliability.
Conclusion
The integration of spam detection using machine learning into IT services and security systems is not just a trend but a necessity in today’s digital landscape. As threats evolve, so must our strategies for combating them. By adopting advanced technological solutions, businesses can protect their sensitive data, maintain customer trust, and ultimately enhance their operational efficiency.
At Spambrella.com, we are committed to providing top-notch IT services and computer repair solutions tailored to meet the unique needs of our clients. Our focus on advanced spam detection methodologies ensures a safer, more productive online environment.
Take the first step towards a spam-free future by embracing machine learning solutions today!